Data Review and Tracking.
The Evolution from Data Integrity to Data Quality
By Divya Ravishankar, Director Product Strategy, ThoughtSphere
July, 2023
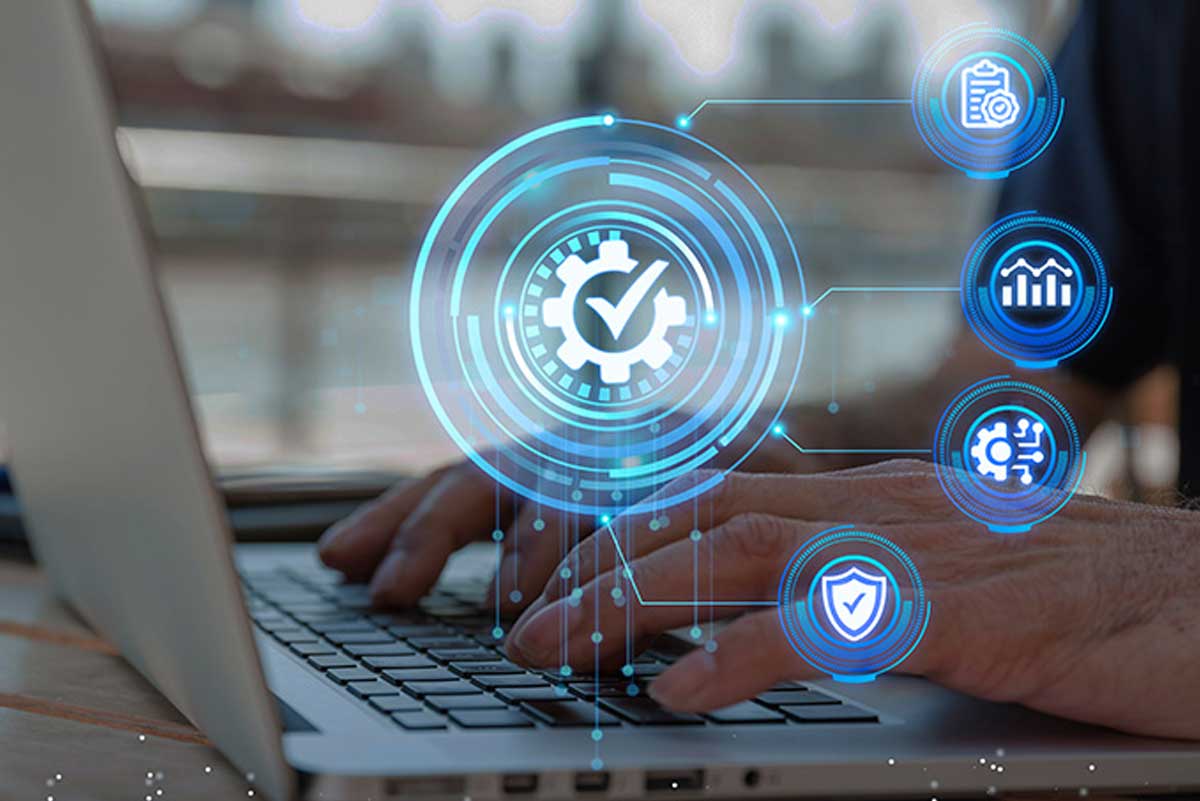
Need to Evolve
Do you remember in the not-so-long ago past, when a typical clinical trial had 2-3 data sources and less than 10 exploratory endpoints? A time when the process of integrating data, albeit needed, was far less complicated and the ALCOA data integrity principles dictated the data cleaning and monitoring strategy?
Well, let’s shift from memory lane and come back to today, where less than 40% of clinical data comes from the eCRF and an average study has 6 or more data sources, many of which are digital, collecting data directly from the patient. In this new world of clinical trials, the traditional, cookie-cutter data cleaning approach of applying data checks, listing reviews, and sending a CRA onsite to monitor data integrity, is not enough. Life Science organizations must incorporate data quality measures across the complete clinical delivery life cycle and invoke automated process flows to monitor data validity and keep pace with data velocity demands.
To support the shift, industry guidance endorsed by the FDA and EMA has emerged for building quality into clinical studies systematically and operationally. ICH E6(R2) and the recently released draft of E6(R3) outline data quality and governance requirements for data security, auditability, traceability, and transformation. Additionally, corresponding guidance is provided in ICH E8(R1) for designing quality into clinical trials and protecting Critical to Quality (CtQ) Factors. These ICH guidelines set the groundwork for the adoption of an outcome driven, risk-based, central monitoring strategy that examines not only the integrity of the data, but also the veracity and reliability of the data across an ever-growing landscape of digital data sources and complex trial designs.
So, what are some steps you can take to transition to a more robust and quality-driven data cleaning and central monitoring strategy? Distilled below are 3 important considerations to keep in mind as you start the journey:
1. Embrace Technology Innovation
A robust central monitoring strategy requires multiple layers of cross-functional reviews to examine the data and distill new insights. To do this and not break the bank or create process bottlenecks, organizations must enable technology to integrate and automate processes from data aggregation to the generation of validation-ready datasets. This provides project teams efficient ways to mine and share operational, clinical and metadata findings to monitor patient safety and ensure data integrity and veracity. Integrated platforms also enable the automation of review workflows and provide full transparency across the data review process to support collaboration and study oversight, hence reducing review cycle times for faster data deliveries.
Advanced capabilities such as automated data change tracking that highlight new or modified data since the last review cycle can also shorten data review timelines. This technology-enabled feature is especially useful when performing comprehensive clinician assessments such as patient profile reviews because it allows medical monitors to easily see and focus on data that has changed since their last assessment.
Lastly, many technology vendors allow organizations to apply proprietary AI/ML models within the platform to further tailor and strengthen their data cleaning processes. Some examples include models for advanced anomaly detection, correlation analyses, signal detection (safety & scientific misconduct) as well as the ability to set study benchmarks and QTLs using historical clinical trial data as well as RWE. By being able to develop and deploy novel models within the platform, this allows organizations to differentiate from competitors and future-proof their technology investment to support translational medicine, advanced genomic trials, as well as further improve operational processes and data surveillance techniques overtime.
2. Take an Agile, Outcome-based QbD Approach:
With patient well-being and statistical analysis in mind, it is important to ensure that Critical-to-Quality (CtQ) Factors have been identified and risks associated with collecting and reporting them are actively mitigated. Not only should the central monitoring strategy encompass the mitigation mechanisms used to control, detect, and/or monitor risks, but it should also allow the study team to work with agility when applying risk control mechanisms throughout the study. Study teams must be able to add, remove and revise data review activities and risk control mechanisms nimbly when unforeseen risks appear and known risks dissipate or heighten in reaction to the dynamics of the study as it progresses from start-up to conduct to close-out. For example, when monitoring subject eligibility risks on a slow recruiting study, the data cleaning strategy should leverage more detection-based, patient level review mechanisms early in study, but switch to more automated, statistical review mechanisms as the study progresses and the volume of subjects and data increases. By taking a focused, outcome-based approach that allows for process agility, you can yield better quality and also improve review efficiency times as the study progresses.
3. Refine your Organization’s Data Strategy:
Last, but definitely not least, be aware of the organizational level data strategy and the decision-makers involved. Every organization, big or small, grapples with defining and implementing an all-encompassing, organizational level data strategy and infrastructure. Then, once implemented It is an ever-evolving task to maintain a data governance framework that scales to shifting business objectives, fosters seamless data integration, and enables technology innovations.
With-that-said, the organizational data strategy goals and IT framework can have a huge impact on what is or is not possible regarding the central monitoring data strategy and often dictates (or heavily influences) the technology choices and processes that underpin a risk-based data review and monitoring strategy. Being aware of this broader perspective, and if possible, having a seat at the table to share central monitoring strategy needs is crucial.
Summary:
As the clinical trial landscape changes with complex trial designs, new regulations, and the ever-growing volume and variety of data, organizations must evolve their data quality and review strategies to encompass new review methods, incorporate automation opportunities, and take a quality by design approach that is outcome driven.
To do this effectively, organizations should consider new technologies available, revamp their operational processes to support an agile outcome-based strategy, and ensure central monitoring strategy goals align with the over-arching organizational data strategy.
Unlock the Potential of Unified Clinical Data with ThoughtSphere!