Are you Aiming for the Bullseye or Just the Target?
Developing an Outcome-based RBQM Strategy
By Christina Dinger, Senior Director of Product, ThoughtSphere
Jun, 2023
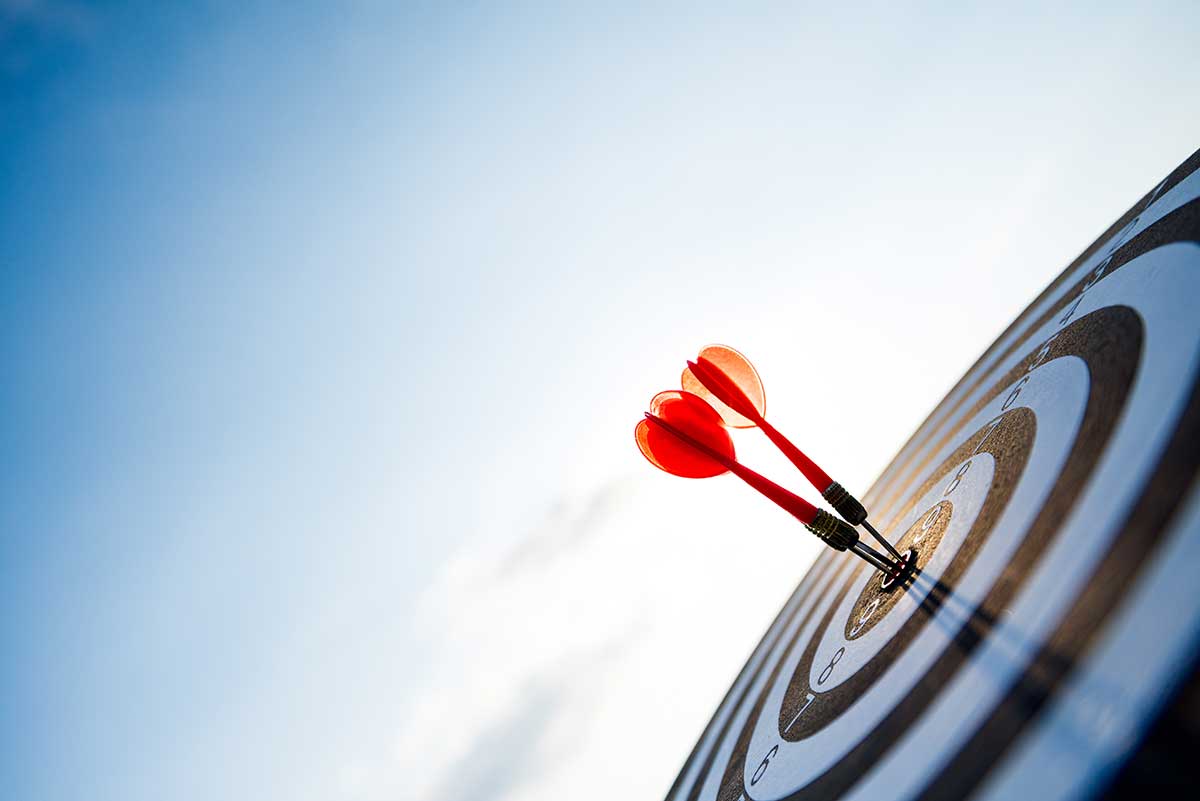
Imagine a life-long friend who you haven’t seen in years is traveling to your area for work. You make plans to meet up in the city where your friend is staying, a suburb of your area, but not a place you go to often. The two of you pick a date, time, and a five-star restaurant that you both want to try. When the day arrives, you open Google Maps to chart your course. Google Maps uses your current location and the restaurant address to provide step-by-step, turn-by-turn directions from your house to the restaurant. You are given the total travel distance, the expected arrival time, and even alerted if you need money for tolls.
Now imagine leaving to meet your friend, but only entering the name of the city into Google Maps. No restaurant name, no address, no additional coordinates, just the city name. Without any other outside aid, how successful do you think you will be in finding the restaurant?
So How Does Google Maps Relate to Clinical Trials?
Well, let’s shift gears (pun intended) and think about the adoption and application of Risk-based Quality Management (RBQM) in clinical trial operations. First, we start with the risk assessment. We review the primary and secondary endpoints, the Time & Events, and other key sections in the protocol and do our best to extract the Critical to Quality (CtQ) Factors. The project team then goes to work identifying, prioritizing, and mitigating risks to protect the CtQ Factors.
While this is a good start, the study’s purpose or target is set and plans to mitigate obstacles from hitting the target are developed, it is not enough. The target’s bull’s eye is never brought into focus. It is usually not until the study is underway and crucial decisions have already been implemented (i.e., CRF designed, Vendor Specifications finalized, Edit Specifications created, etc.) that the Statistical Analysis Plan (SAP) is drafted. It is even later in the trial when the tabulation datasets and tables described in the SAP are formulated.
Using the Google Maps illustration as an analogy, consider the statistical analysis deliverables as the final destination; they are the bull’s eye for which we should set our aim. Without them, we may know the major thoroughfares to get us to the destination city and obstacles along the way, but we don’t know the city streets or address to navigate to the restaurant.
All too often this is how clinical trials are executed. We set off on the clinical trial journey, but the lack of precision on where we’re headed leaves a lot of room for error and lack of trust in the RBQM process. So how do we avoid this? How can we take an outcome-based RBQM approach with confidence? Well, the answer requires knowing, with precision, the end deliverable. The protocol is our starting point, and the statistical analysis is our end point. If we have these 2 coordinates clearly defined, identifying the CtQ Factors is easier and developing a well-defined, outcome-based strategy is achievable. Below are a few strategic steps to ensure study operations are aligned and calibrated throughout the study with the statistical analysis deliverables.
Greater Upfront Involvement of the Biostatistician
During study planning and start-up, CtQ Factors should be thoroughly evaluated by the biostatistics team and whenever possible descriptive text provided to specify how each CtQ Factor will be used in the analysis. Covariates that have an impact on CtQ Factors should also be called out for team awareness so that they are adequately collected and reviewed to ensure their reliability and completeness. Stratification factors and other study population attributes that support descriptive analytics and provide study context must also be highlighted to the broader team as they are easy to miss or de-prioritize during the risk assessment. Not only will this collaborative exercise help the biostatistician(s) draft a higher quality SAP, but it will provide the operational team with the significance of data points collected to strengthen the risk assessment output.
Generate Analysis and Tabulation Datasets Early
Clear visibility to how CtQ Factors will be used in the analysis is essential to honing the trial’s aim. Teams should understand, with precision, how CtQ Factors will be used in calculations and presented in datasets, tables, figures, and listings (TFLs) to ensure they are reviewed and interpreted similarly throughout trial conduct leaving no room for surprises at the time of data delivery. This involves mapping the CtQ Factors to tabulation datasets early in the study and using the transformed data to support operational tools and data reviews where appropriate. This also includes knowing factors that will exclude a patient from a study analysis and setting up review mechanisms to quickly identify these patients throughout the study.
Perform Periodic TFL Dry Runs
Performing TFL dry runs sooner in a study (not just prior to Database Lock) is a good step to demonstrate the effectiveness of the data controls put in place and identify where further calibration is needed. For blinded studies, dummy treatment arms with random patient assignments can be used to maintain the blind, but still give visibility to the completeness of data, skewed derivations, and outliers. This supports the identification of protocol deviations, and the significance covariates can have on the analysis.
While some studies have interim safety and Data Monitoring Committee (DMCs) charters that require the delivery of certain TFLs, the trial data is often unblinded and therefore only visible by a select group of non-study team members. Additionally, the full TFL set are typically not produced, only a partial subset, so potential findings and anomalies can slip through the cracks. Thus, it is recommended to generate TFLs from an outcome-based RBM strategy perspective. This allows the team to gauge the quality of the data and refine risk control mechanisms and data review activities throughout the trial.
Conclusion:
As an industry that is always looking to reduce costs, save time, and yield high quality, sometimes our pursuit to get a study started overshadows the importance of pinpointing the ultimate deliverable. Thus, we start off towards the study’s target, with poorly calibrated tools and risk controls which leave a margin of uncertainty to the reliability of trial results. By taking a few additional, but strategic steps to align to the statistical analysis and calibrate our aim throughout the study, the target area gets smaller, so even if we don’t hit the bull’s eye dead on, we gain confidence in the process and yield higher accuracy.
Unlock the Potential of Unified Clinical Data with ThoughtSphere!