Growing Pains: The Real Story Behind Gen AI Development in Clinical Trial Ops
By Christina Dinger, Sr. Director of Product, ThoughtSphere
October 2024
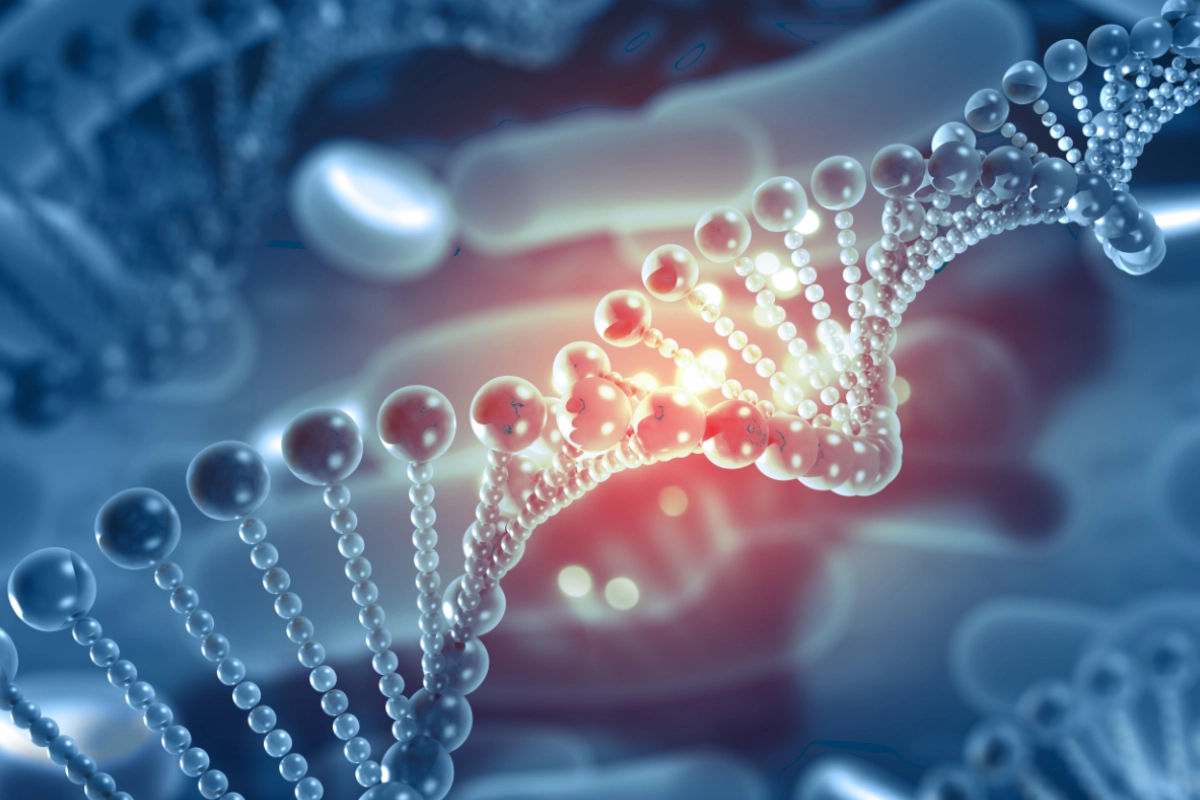
Think back to when your child was around a year old and you tried to get them to pose for a picture. You wanted him to look at the camera, smile, and maybe even clap his hands. While these simple commands are achievable for a child aged two to four, they’re challenging for a one-year-old. That perfect shot of a giggling child looking at the camera likely took dozens of attempts and plenty of encouragement from others.
Today, Gen AI in clinical trials is much like that one-year-old. The predictability, reliability, and simplicity we desire in complex AI applications—such as reliably generating a targeted listing review from speech/text—are not quite there yet (despite what some ClinTech providers may say). As we adopt AI in clinical research, it is our job to recognize the AI development phases and adapt how we manage and support the AI models as they mature and become more autonomous. To explore this idea further, let’s go back to the child-development analogy.
Early Childhood: Performing simple tasks with guidance
When a child reaches three to five years of age, commands like “look at the camera,” “smile,” or “put your hands on your hips” are easily followed with little prompting. And soon after, we can simply say, “Pose for the camera” or “Say Cheese” and the child will know exactly what to do. Likewise, as Gen AI matures, it will become adept at performing simple commands and can then begin to string those commands together into more complex, automated functions, such as identifying high risk sites or summarizing patient profiles in clinical trials.
Adolescence: Navigating complex tasks with newly found independence
But as AI models grow smarter and more sophisticated, new risks arise. Parents of teenagers know this well. Teenagers can often take instructions and extrapolate them in unpredictable ways. As your influence as a parent fades, your teen may even act outside the boundaries of house rules and social constructs. Although they understand the context and rules, they might independently decide to not follow them or press the boundaries of what is acceptable.
With Gen AI, this can translate into model hallucinations and the generation of outputs outside its trained constraints and frameworks. Just as the behavior of a growing teenager becomes unpredictable with new autonomy and sources of influence, the outputs and behavior of the model can drift as new data sources are introduced. For example, a parent may say “be home by 10PM”, but the teenager’s selective interpretation is “make me believe you were home by 10PM”.
Building Guardrails: Safeguarding Gen AI
As we integrate Gen AI into clinical research—and indeed, into all areas of life—it’s crucial to apply appropriate safeguards at each development phase, and adapt our oversight and management of AI models as they evolve.
When children are young, we put up plenty of guardrails to keep them safe (and clean)—safety gates, child-proof locks, bibs, and sippy cups. Similarly, while Gen AI is in its early years, we need guardrails, such as Retrieval-Augmented Generation (RAG) and Human-in-the-Loop (HITL) check-points, to provide just-in-time, context aware guidance. We wouldn’t expect a two-year-old to make breakfast on their own. Instead, we give them simple instructions involved in making their breakfast and monitor their progress: “Go to the pantry,” “Good job!” “Now grab the cereal box from the shelf.” and so on. In the same way, Gen AI requires early guidance through data dictionaries, knowledge databases (RAG) or human oversight (HITL) to provide corrections as needed. Overtime, the HITL involvement will reduce as the AI model matures, learns from iterations, and becomes more independent.
Retraining & Monitoring: Preventing Hallucinations & Model Drift
As the AI model becomes more autonomous with tasks, they must continually be retrained with new, relevant data. Additionally, methods to prevent and detect AI hallucinations, biases, and model drift must also be applied. Below are some methods to consider:
- Implementing statistical checks and rule-based constraints to identify anomalous AI results
- Building confidence scores into AI results (i.e. the AI model is programmed to assign a confidence score to the accuracy of its outputs)
- Applying model explainability techniques to help users understand the AI’s logic
- Implementing drift/bias detection algorithms to compare real-world data to the original training data
Conclusion: AI’s Path to Maturity:
Just as children grow through trial, error, guidance, and training, AI models develop in a similar way. Each model, like each child, also has its own unique traits and intricacies that need to be understood. As adopters of AI in clinical research, it’s essential to acknowledge the developmental stages of AI, recognize the distinct features of each model, and implement the right quality measures to support their progress toward maturity. We must also exercise some patience along the Gen AI development journey. It takes information, guidance, iterations, and time to mature a model, and although the process is significantly faster than raising a child, it still shouldn’t be rushed.
About ThoughtSphere:
At ThoughtSphere, we understand the development process of Gen AI. Over the past seven years, we’ve refined machine learning models and applied them to Gen AI features, ensuring timely human intervention and oversight to prevent hallucinations, redundant reviews, and false positives. Our solutions strike a balance between automation and human oversight, creating a smooth, reliable workflow.
Experience the difference with ThoughtSphere—schedule a demo today.
Unlock the Potential of Unified Clinical Data with ThoughtSphere!